The past twenty years have seen vast strides forward in developing electron, X-ray, and neutron diffraction-based characterizations that probe engineering alloys during in-situ processing and thermo-mechanical loading at increasingly short time scales. However, the full utility of these experiments is limited in that the analyses of the measured data extract only a fraction of the encoded information about the microstructure at any given time. Rather than analyze diffraction data with a physics-based X-ray model to try to extract structural information chosen a priori, a team comprised of researchers from CHEXS, Cornell, and NIST used the unsupervised learning technique, locally linear embedding (LLE), to condense X-ray data down to critical microstructural (dislocation configuration) evolution information. The LLE method finds a set of coordinates in a lower-dimensional space that maintains the local neighborhood character (similarity or dissimilarity) of nearby data observations. While LLE will determine similarity in a tractable representation, physical understanding still comes from discovering what underlying features or physical processes maintains the neighborhood character.
The research team applied LLE directly to in-situ X-ray diffraction data to discover and monitor underlying dislocation motion in an additively manufactured (AM) nickel-based superalloy, Inconel 625 (IN625). They find that in this case, the LLE analysis of time series X-ray diffraction data identifies, without intervention, both the onset of plastic deformation (yield) and transients associated with the mobility of dislocation populations that underlie the strength and plasticity behavior in the alloy. While this is a single demonstration, the approach can be applied to numerous other studies of deformation and materials processing.
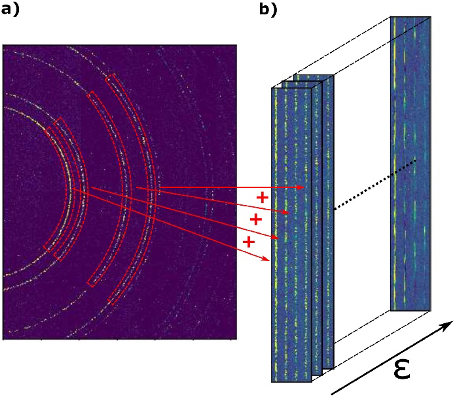
Broader Impacts
The application of various machine learning techniques to materials science has promised to revolutionize the field, but to date, application to structural materials performance or processing has been limited. Researchers foresee the approach demonstrated here being applied to many other data types and material evolution conditions beyond X-ray diffraction data and uniaxial loading. Any time series of scattering data (electrons, neutrons, or X-rays) encoded with microstructural information will likely yield valuable insight, and the approach can also be applied to data gathered during other thermo-mechanical loading conditions and processing environments. A natural application is the analysis of diffraction data measured during additive manufacturing processes or other extreme heating and cooling scenarios, such as quenching, to identify critical points of microstructural development and guide modeling of kinetics in constitutive models. However, even applying this analysis to understanding more traditional heat treatments for process modeling optimization could prove extremely valuable.
Need for CHEXS Experimental Capabilities
The full cross section of the AM IN625 specimen was illuminated by a high-energy X-ray beam (61.332 keV) at CHESS, one of the few facilities in the world optimized for this type of experiment. The X-ray beam, which was 1 mm tall by 2 mm wide, illuminated a volume at the center of the gauge section and traveled in the –z direction. As the sample was deformed, the sample was also continuously rotated (rocked) about the y direction to rotate more crystals into the diffraction condition. Diffraction peaks from a 10○ rocking motion were integrated onto single images for processing. The diffraction data was collected on a Dexela 2923 area detector located 877 mm behind the specimen every 470 s. The detector was translated horizontally 135 mm from the direct X-ray beam to prevent collection of diffraction peaks near the rotation axis that would provide misleading characterization of the microstructure evolution.
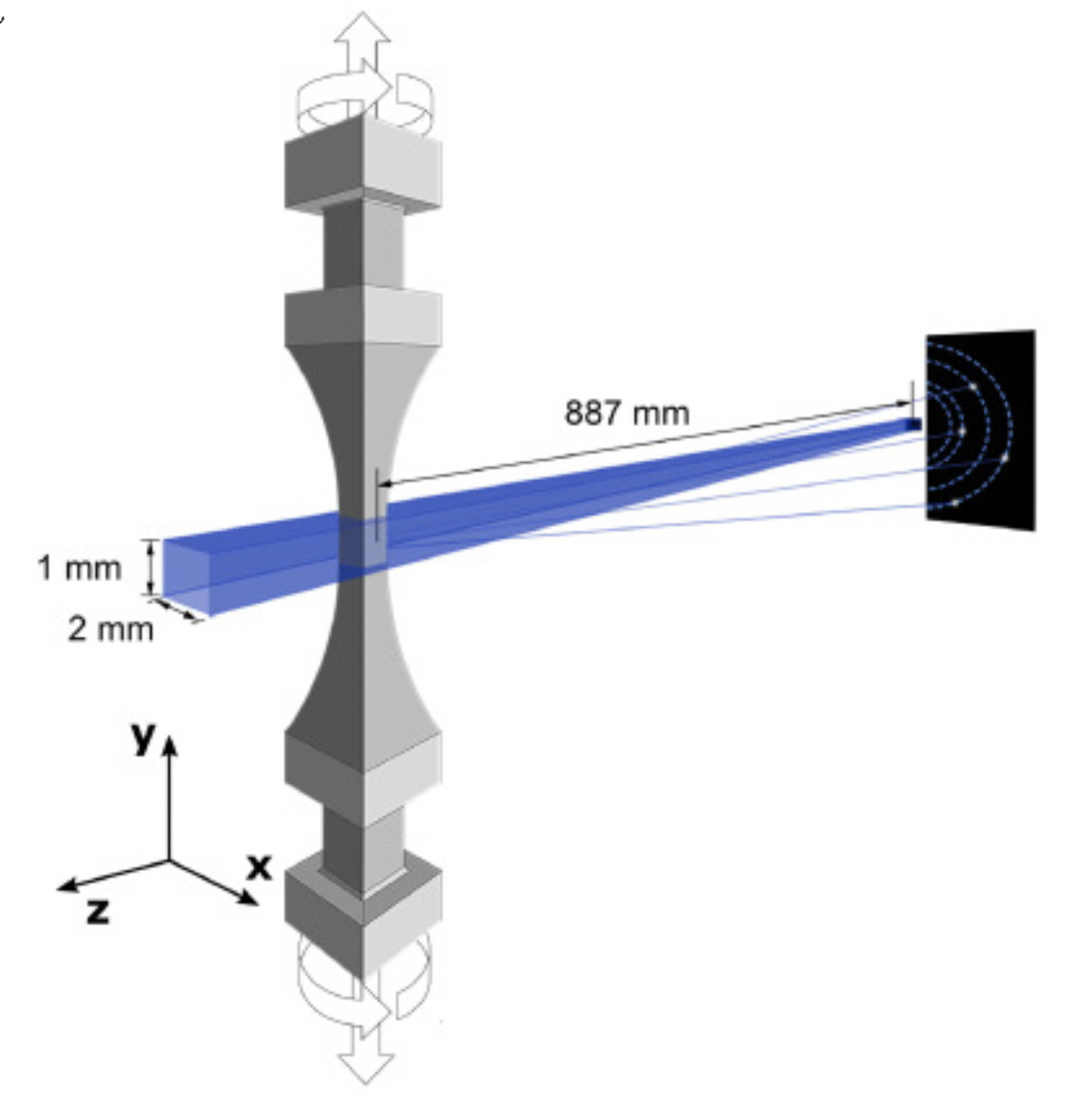
Funding
This work is based upon research conducted at the Center for High Energy X-ray Sciences (CHEXS) which is supported by the National Science Foundation under award DMR-1829070. AJB received support through the Office of Naval Research (Contract N00014-16-1-3126).
Reference:
Darren C. Pagan, Thien Q. Phan, Jordan S. Weaver, Austin R. Benson, and Armand J. Beaudoin
Unsupervised learning of dislocation motion
Acta Materialia 181, 510 (2019)