What is the discovery?
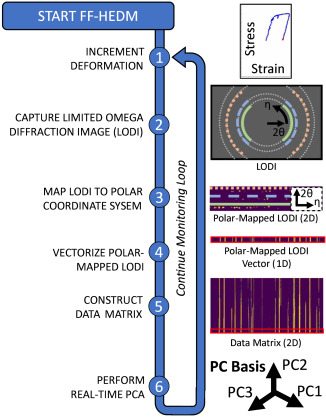
High energy diffraction microscopy (HEDM) techniques offer the only means of measuring grain-scale fatigue in metals in three-dimensions and under loading and heating conditions. Far-field HEDM (ff-HEDM) studies at the FAST (Forming and Shaping Technology) beamline at CHEXS can probe the thousands of individual grains within a polycrystalline metal to understand the micromechanics and microstructural response of each grain within its unique local environment. However, ff-HEDM is limited by data collection rates (typically > 5 minutes to collect a full dataset) and a complex data analysis process which typically takes even longer. Now, a new paper appearing in the International Journal of Fatigue reports the first implementation of a real-time data analysis method for ff-HEDM, which can extract meaningful microscopic information as measurements are collected. The method relies on rapidly analyzing partial subsets of the data, using <4% of the detection channels and <1% of the sample rotation range of a typical HEDM dataset. The team consisting of lead author graduate student Dalton Shadle, and Cornell Engineering faculty members Matt Miller and Kelly Nygren, used principal component analysis (PCA) on these partial datasets (which include signal from a small number of grains) and demonstrated that the first 3 components can be correlated with material deformation processes, which can be targeted for further study with full ff-HEDM measurements. This builds on earlier demonstrations at CHEXS of unsupervised machine learning for dimensional reduction of HEDM data, although those methods were not implemented live during experiments. The new analysis method can be performed as quickly as the data can be collected (many data points each second), offering truly real-time, microscopic feedback for users of the FAST beamline.
Why is it important?
Complex multi-dimensional diffraction studies with high energy x-rays are a core mission of the two most over-subscribed beamlines at CHEXS (the FAST and QM2 beamlines). However, these precision measurements generate TBs of information-rich data and often require weeks (or even months) to fully understand. This means that CHEXS users may only learn what really is happening inside their sample long after the (typically 2-6 day) experiment is complete. A key long-term goal of the facility is to develop ways to understand “big data” from these experiments in real time, to empower the users to make informed decisions and optimize the chances for success. The work of Shadle, Miller, and Nygren demonstrates a practical implementation that promises to immediately improve the success rate of many FAST users and inspire similar methods to be implemented at other beamlines.
Why did this research need CHEXS?
The FAST beamline at CHEXS is dedicated to understanding engineering materials processing and performance. FAST is the premier facility worldwide for HEDM measurements, which were used for this work. These measurements require the high flux of high energy x-rays which are available at FAST, as well as the suite of in-house detectors and custom load-frames provided to users. This research also made use of the advanced computational infrastructure (both hardware and software) available at FAST to tackle the “big data” challenges associated with rapidly analyzing information from thousands of crystalline grains.
How was the work funded?
Center for High Energy X-ray Sciences (CHEXS), National Science Foundation (DMR-1829070)
US Dept of Energy, Basic Energy Sciences, Grant No. DE-SC0018901
Reference:
Using real-time data analysis to conduct next-generation synchrotron fatigue studies
DJ Shadle, MP Miller, and KE Nygren
International Journal of Fatigue 164 (2022) 107113; https://doi.org/10.1016/j.ijfatigue.2022.107113